ARTICLE AD BOX
An international research team has developed a new benchmark that reveals the current limitations of LLMs. Even the most advanced models fail at 90 percent of the tasks - for now.
The test, called "Humanity's Last Exam" (HLE), includes 3,000 questions across more than 100 specialized fields, with 42 percent focusing on mathematics. Nearly 1,000 experts from 500 institutions across 50 countries contributed to its development.
The researchers started with 70,000 questions and presented them to leading AI models. Of these, 13,000 questions proved too difficult for the AI systems. These questions were then refined and reviewed by human experts, who were paid between $500 and $5,000 for high-quality contributions. 3,000 questions made it into the dataset.
The results aren't flattering. Even the most sophisticated AI models struggle on this benchmark. GPT-4o solves only 3.3 percent of the tasks correctly, while OpenAI's o1 achieves 9.1 percent and Gemini 6.2 percent. The difficulty of the test is partly by design, as only questions that initially stumped these AI models were included in the final version.
Ad
THE DECODER Newsletter
The most important AI news straight to your inbox.
✓ Weekly
✓ Free
✓ Cancel at any time
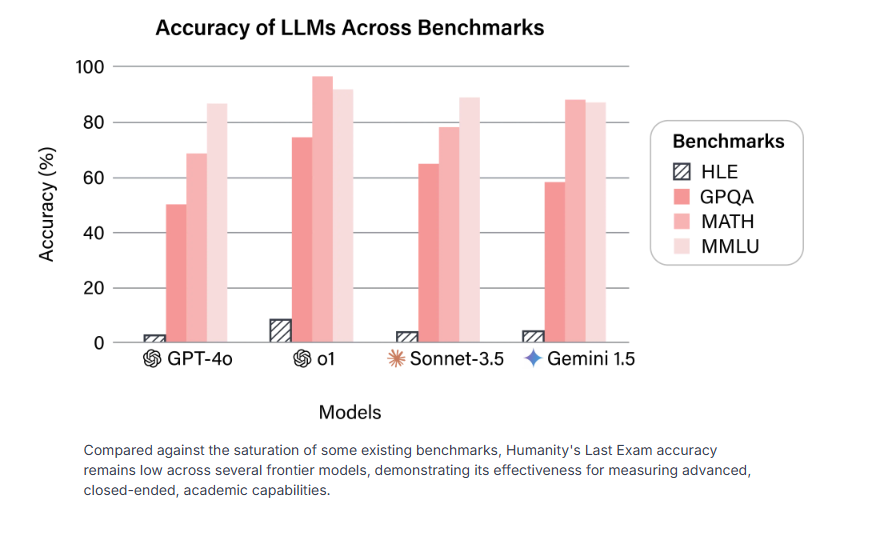
One of the more troubling findings is how poorly AI systems assess their own capabilities. Models show extreme overconfidence, with calibration errors exceeding 80 percent - meaning they're usually very sure of their wrong answers. This disconnect between confidence and accuracy makes working with generative AI systems particularly challenging.
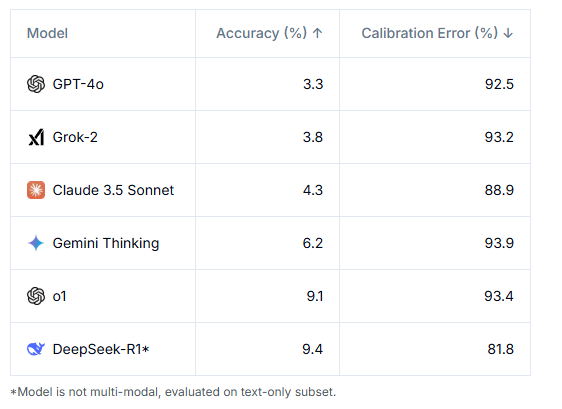
The project emerged from a collaboration between the Center for AI Safety and Scale AI. Dan Hendrycks, who leads the Center for AI Safety and advises Elon Musk's xAI startup, spearheaded the initiative.
Experts push back on the "final test" concept
Not everyone supports this academic approach to AI evaluation. Subbarao Kambhampati, former president of the Association for the Advancement of Artificial Intelligence, argues that humanity's essence isn't captured by a static test but rather by our ability to evolve and tackle previously unimaginable questions. AI expert Niels Rogge shares this skepticism, suggesting that memorizing obscure facts isn't as valuable as developing practical capabilities.
Former OpenAI developer Andrej Karpathy notes that while academic benchmarks are easy to create and measure, testing truly important AI capabilities - like solving complex, real-world problems - proves much more challenging.
He sees these academic benchmark results as a new version of Moravec's paradox: AI systems can excel at complex rule-based tasks like math, but often struggle with simple problems that humans solve effortlessly. Karpathy argues that testing how well an AI system could function as an intern would be more useful for measuring general AI progress than its ability to answer academic questions.
Recommendation
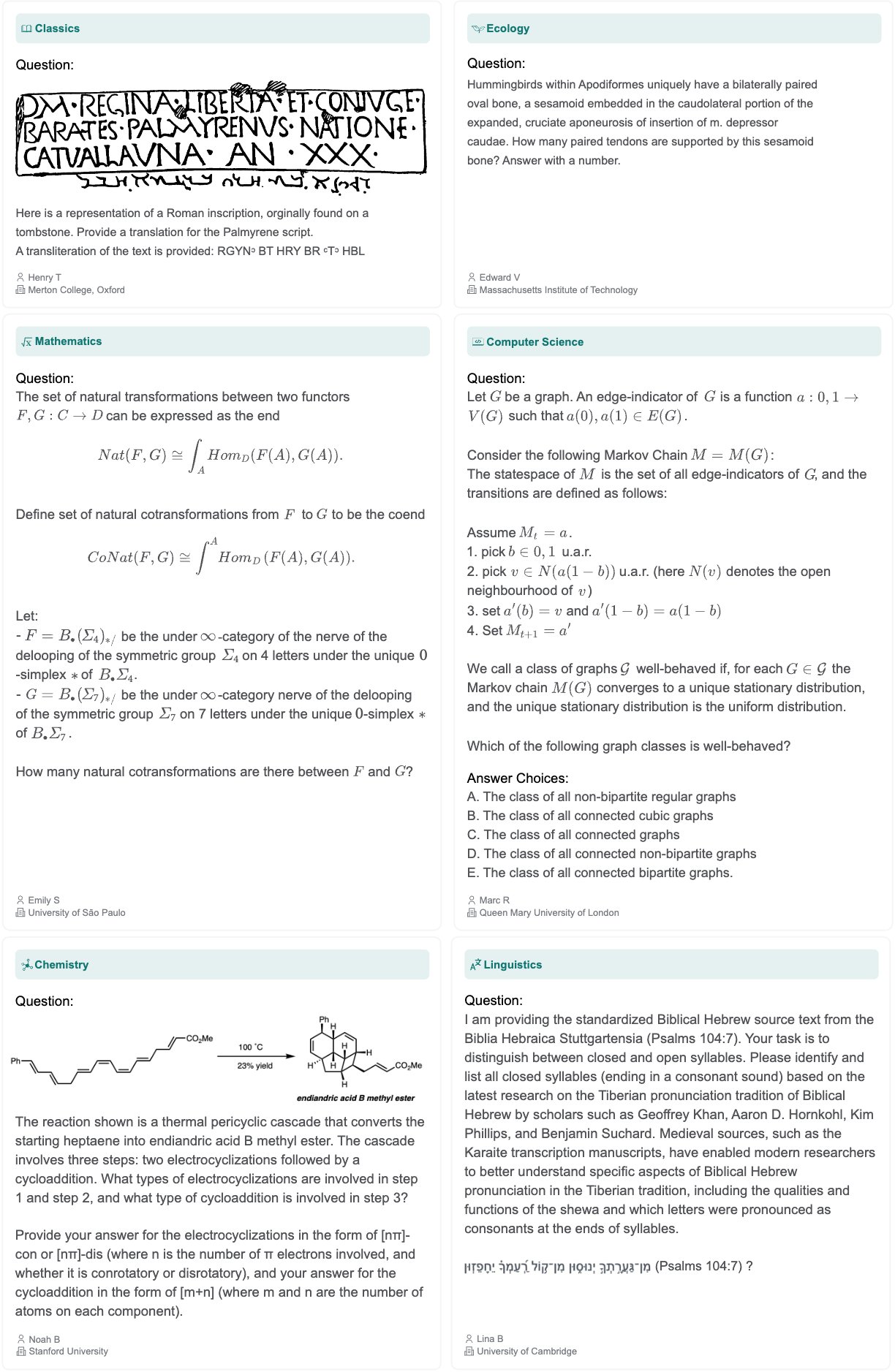
The benchmark's developers predict AI systems will correctly answer more than 50 percent of HLE questions by late 2025. However, they themselves warn against premature interpretations.
Even if AI models reach this milestone, it wouldn't prove the existence of artificial general intelligence. The test assesses expert knowledge and scientific understanding, but only through structured academic problems - it doesn't evaluate open research questions or creative problem-solving abilities.
As Kevin Zhou from UC Berkeley points out to The New York Times, "There’s a big gulf between what it means to take an exam and what it means to be a practicing physicist and researcher. Even an A.I. that can answer these questions might not be ready to help in research, which is inherently less structured."
The test's real value might be helping scientists and policymakers better understand AI capabilities, providing concrete data for discussions about development, risks, and regulation.